Ministry is an adventure. It's time to start navigating it better. This podcast focuses on doing better ministry. You'll find thoughts and perspectives on effective ministry from 2 decades of ministry consulting.
…
continue reading
The Multi-Award Garbedian on Golf is the most comprehensive, entertaining & informative Golf Talk Radio Program available to Avid Golfers of All Kinds. Let's Tee it Up!
…
continue reading
AXRP (pronounced axe-urp) is the AI X-risk Research Podcast where I, Daniel Filan, have conversations with researchers about their papers. We discuss the paper, and hopefully get a sense of why it's been written and how it might reduce the risk of AI causing an existential catastrophe: that is, permanently and drastically curtailing humanity's future potential. You can visit the website and read transcripts at axrp.net.
…
continue reading
Home of the Hero Support Records Pod Network!! The Punk Rock Movie Discussion! - Punk People from Punk Things talking movies!! As a musician in the punk scene.. you meet lots of musicians and music types.. Dewayne Burns (Hero Support Records) gabs with fellow musicians and such about their favorite flicks.. because why would musicians talk about music...Find all the Hero Support band releases at >>www.herosupport.bandcamp.com << Podcast theme - Sleeping Bag by Get Bent
…
continue reading
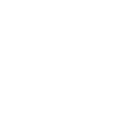
1
40 - Jason Gross on Compact Proofs and Interpretability
2:36:05
2:36:05
Play later
Play later
Lists
Like
Liked
2:36:05How do we figure out whether interpretability is doing its job? One way is to see if it helps us prove things about models that we care about knowing. In this episode, I speak with Jason Gross about his agenda to benchmark interpretability in this way, and his exploration of the intersection of proofs and modern machine learning. Patreon: https://w…
…
continue reading
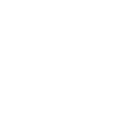
1
38.8 - David Duvenaud on Sabotage Evaluations and the Post-AGI Future
20:42
20:42
Play later
Play later
Lists
Like
Liked
20:42In this episode, I chat with David Duvenaud about two topics he's been thinking about: firstly, a paper he wrote about evaluating whether or not frontier models can sabotage human decision-making or monitoring of the same models; and secondly, the difficult situation humans find themselves in in a post-AGI future, even if AI is aligned with human i…
…
continue reading
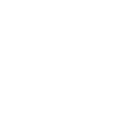
1
38.7 - Anthony Aguirre on the Future of Life Institute
22:39
22:39
Play later
Play later
Lists
Like
Liked
22:39The Future of Life Institute is one of the oldest and most prominant organizations in the AI existential safety space, working on such topics as the AI pause open letter and how the EU AI Act can be improved. Metaculus is one of the premier forecasting sites on the internet. Behind both of them lie one man: Anthony Aguirre, who I talk with in this …
…
continue reading
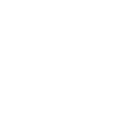
1
38.6 - Joel Lehman on Positive Visions of AI
15:28
15:28
Play later
Play later
Lists
Like
Liked
15:28Typically this podcast talks about how to avert destruction from AI. But what would it take to ensure AI promotes human flourishing as well as it can? Is alignment to individuals enough, and if not, where do we go form here? In this episode, I talk with Joel Lehman about these questions. Patreon: https://www.patreon.com/axrpodcast Ko-fi: https://ko…
…
continue reading
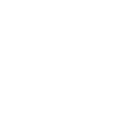
1
38.5 - Adrià Garriga-Alonso on Detecting AI Scheming
27:41
27:41
Play later
Play later
Lists
Like
Liked
27:41Suppose we're worried about AIs engaging in long-term plans that they don't tell us about. If we were to peek inside their brains, what should we look for to check whether this was happening? In this episode Adrià Garriga-Alonso talks about his work trying to answer this question. Patreon: https://www.patreon.com/axrpodcast Ko-fi: https://ko-fi.com…
…
continue reading
AI researchers often complain about the poor coverage of their work in the news media. But why is this happening, and how can it be fixed? In this episode, I speak with Shakeel Hashim about the resource constraints facing AI journalism, the disconnect between journalists' and AI researchers' views on transformative AI, and efforts to improve the st…
…
continue reading
Lots of people in the AI safety space worry about models being able to make deliberate, multi-step plans. But can we already see this in existing neural nets? In this episode, I talk with Erik Jenner about his work looking at internal look-ahead within chess-playing neural networks. Patreon: https://www.patreon.com/axrpodcast Ko-fi: https://ko-fi.c…
…
continue reading
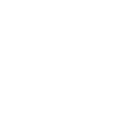
1
39 - Evan Hubinger on Model Organisms of Misalignment
1:45:47
1:45:47
Play later
Play later
Lists
Like
Liked
1:45:47The 'model organisms of misalignment' line of research creates AI models that exhibit various types of misalignment, and studies them to try to understand how the misalignment occurs and whether it can be somehow removed. In this episode, Evan Hubinger talks about two papers he's worked on at Anthropic under this agenda: "Sleeper Agents" and "Sycop…
…
continue reading
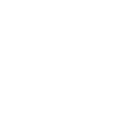
1
38.2 - Jesse Hoogland on Singular Learning Theory
18:18
18:18
Play later
Play later
Lists
Like
Liked
18:18You may have heard of singular learning theory, and its "local learning coefficient", or LLC - but have you heard of the refined LLC? In this episode, I chat with Jesse Hoogland about his work on SLT, and using the refined LLC to find a new circuit in language models. Patreon: https://www.patreon.com/axrpodcast Ko-fi: https://ko-fi.com/axrpodcast T…
…
continue reading
Road lines, street lights, and licence plates are examples of infrastructure used to ensure that roads operate smoothly. In this episode, Alan Chan talks about using similar interventions to help avoid bad outcomes from the deployment of AI agents. Patreon: https://www.patreon.com/axrpodcast Ko-fi: https://ko-fi.com/axrpodcast The transcript: https…
…
continue reading
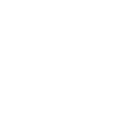
1
38.0 - Zhijing Jin on LLMs, Causality, and Multi-Agent Systems
22:42
22:42
Play later
Play later
Lists
Like
Liked
22:42Do language models understand the causal structure of the world, or do they merely note correlations? And what happens when you build a big AI society out of them? In this brief episode, recorded at the Bay Area Alignment Workshop, I chat with Zhijing Jin about her research on these questions. Patreon: https://www.patreon.com/axrpodcast Ko-fi: http…
…
continue reading
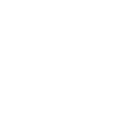
1
37 - Jaime Sevilla on AI Forecasting
1:44:25
1:44:25
Play later
Play later
Lists
Like
Liked
1:44:25Epoch AI is the premier organization that tracks the trajectory of AI - how much compute is used, the role of algorithmic improvements, the growth in data used, and when the above trends might hit an end. In this episode, I speak with the director of Epoch AI, Jaime Sevilla, about how compute, data, and algorithmic improvements are impacting AI, an…
…
continue reading
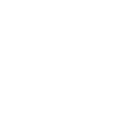
1
36 - Adam Shai and Paul Riechers on Computational Mechanics
1:48:27
1:48:27
Play later
Play later
Lists
Like
Liked
1:48:27Sometimes, people talk about transformers as having "world models" as a result of being trained to predict text data on the internet. But what does this even mean? In this episode, I talk with Adam Shai and Paul Riechers about their work applying computational mechanics, a sub-field of physics studying how to predict random processes, to neural net…
…
continue reading
Patreon: https://www.patreon.com/axrpodcast MATS: https://www.matsprogram.org Note: I'm employed by MATS, but they're not paying me to make this video.
…
continue reading
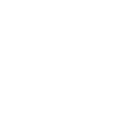
1
35 - Peter Hase on LLM Beliefs and Easy-to-Hard Generalization
2:17:24
2:17:24
Play later
Play later
Lists
Like
Liked
2:17:24How do we figure out what large language models believe? In fact, do they even have beliefs? Do those beliefs have locations, and if so, can we edit those locations to change the beliefs? Also, how are we going to get AI to perform tasks so hard that we can't figure out if they succeeded at them? In this episode, I chat with Peter Hase about his re…
…
continue reading
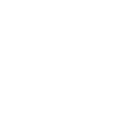
1
34 - AI Evaluations with Beth Barnes
2:14:02
2:14:02
Play later
Play later
Lists
Like
Liked
2:14:02How can we figure out if AIs are capable enough to pose a threat to humans? When should we make a big effort to mitigate risks of catastrophic AI misbehaviour? In this episode, I chat with Beth Barnes, founder of and head of research at METR, about these questions and more. Patreon: patreon.com/axrpodcast Ko-fi: ko-fi.com/axrpodcast The transcript:…
…
continue reading
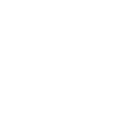
1
33 - RLHF Problems with Scott Emmons
1:41:24
1:41:24
Play later
Play later
Lists
Like
Liked
1:41:24Reinforcement Learning from Human Feedback, or RLHF, is one of the main ways that makers of large language models make them 'aligned'. But people have long noted that there are difficulties with this approach when the models are smarter than the humans providing feedback. In this episode, I talk with Scott Emmons about his work categorizing the pro…
…
continue reading
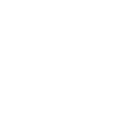
1
32 - Understanding Agency with Jan Kulveit
2:22:29
2:22:29
Play later
Play later
Lists
Like
Liked
2:22:29What's the difference between a large language model and the human brain? And what's wrong with our theories of agency? In this episode, I chat about these questions with Jan Kulveit, who leads the Alignment of Complex Systems research group. Patreon: patreon.com/axrpodcast Ko-fi: ko-fi.com/axrpodcast The transcript: axrp.net/episode/2024/05/30/epi…
…
continue reading
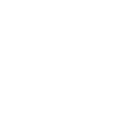
1
31 - Singular Learning Theory with Daniel Murfet
2:32:07
2:32:07
Play later
Play later
Lists
Like
Liked
2:32:07What's going on with deep learning? What sorts of models get learned, and what are the learning dynamics? Singular learning theory is a theory of Bayesian statistics broad enough in scope to encompass deep neural networks that may help answer these questions. In this episode, I speak with Daniel Murfet about this research program and what it tells …
…
continue reading
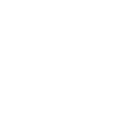
1
30 - AI Security with Jeffrey Ladish
2:15:44
2:15:44
Play later
Play later
Lists
Like
Liked
2:15:44Top labs use various forms of "safety training" on models before their release to make sure they don't do nasty stuff - but how robust is that? How can we ensure that the weights of powerful AIs don't get leaked or stolen? And what can AI even do these days? In this episode, I speak with Jeffrey Ladish about security and AI. Patreon: patreon.com/ax…
…
continue reading
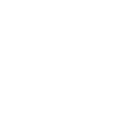
1
29 - Science of Deep Learning with Vikrant Varma
2:13:46
2:13:46
Play later
Play later
Lists
Like
Liked
2:13:46In 2022, it was announced that a fairly simple method can be used to extract the true beliefs of a language model on any given topic, without having to actually understand the topic at hand. Earlier, in 2021, it was announced that neural networks sometimes 'grok': that is, when training them on certain tasks, they initially memorize their training …
…
continue reading
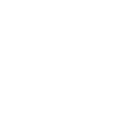
1
28 - Suing Labs for AI Risk with Gabriel Weil
1:57:30
1:57:30
Play later
Play later
Lists
Like
Liked
1:57:30How should the law govern AI? Those concerned about existential risks often push either for bans or for regulations meant to ensure that AI is developed safely - but another approach is possible. In this episode, Gabriel Weil talks about his proposal to modify tort law to enable people to sue AI companies for disasters that are "nearly catastrophic…
…
continue reading
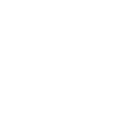
1
27 - AI Control with Buck Shlegeris and Ryan Greenblatt
2:56:05
2:56:05
Play later
Play later
Lists
Like
Liked
2:56:05A lot of work to prevent AI existential risk takes the form of ensuring that AIs don't want to cause harm or take over the world---or in other words, ensuring that they're aligned. In this episode, I talk with Buck Shlegeris and Ryan Greenblatt about a different approach, called "AI control": ensuring that AI systems couldn't take over the world, e…
…
continue reading
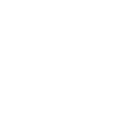
1
26 - AI Governance with Elizabeth Seger
1:57:13
1:57:13
Play later
Play later
Lists
Like
Liked
1:57:13The events of this year have highlighted important questions about the governance of artificial intelligence. For instance, what does it mean to democratize AI? And how should we balance benefits and dangers of open-sourcing powerful AI systems such as large language models? In this episode, I speak with Elizabeth Seger about her research on these …
…
continue reading
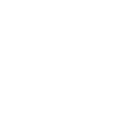
1
25 - Cooperative AI with Caspar Oesterheld
3:02:09
3:02:09
Play later
Play later
Lists
Like
Liked
3:02:09Imagine a world where there are many powerful AI systems, working at cross purposes. You could suppose that different governments use AIs to manage their militaries, or simply that many powerful AIs have their own wills. At any rate, it seems valuable for them to be able to cooperatively work together and minimize pointless conflict. How do we ensu…
…
continue reading
Recently, OpenAI made a splash by announcing a new "Superalignment" team. Lead by Jan Leike and Ilya Sutskever, the team would consist of top researchers, attempting to solve alignment for superintelligent AIs in four years by figuring out how to build a trustworthy human-level AI alignment researcher, and then using it to solve the rest of the pro…
…
continue reading
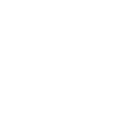
1
23 - Mechanistic Anomaly Detection with Mark Xu
2:05:52
2:05:52
Play later
Play later
Lists
Like
Liked
2:05:52Is there some way we can detect bad behaviour in our AI system without having to know exactly what it looks like? In this episode, I speak with Mark Xu about mechanistic anomaly detection: a research direction based on the idea of detecting strange things happening in neural networks, in the hope that that will alert us of potential treacherous tur…
…
continue reading
Very brief survey: bit.ly/axrpsurvey2023 Store is closing in a week! Link: store.axrp.net/ Patreon: patreon.com/axrpodcast Ko-fi: ko-fi.com/axrpodcast
…
continue reading
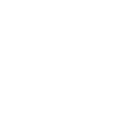
1
22 - Shard Theory with Quintin Pope
3:28:21
3:28:21
Play later
Play later
Lists
Like
Liked
3:28:21What can we learn about advanced deep learning systems by understanding how humans learn and form values over their lifetimes? Will superhuman AI look like ruthless coherent utility optimization, or more like a mishmash of contextually activated desires? This episode's guest, Quintin Pope, has been thinking about these questions as a leading resear…
…
continue reading
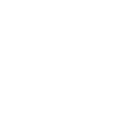
1
Episode 4: Nostalgia might be hurting your ministry
10:24
10:24
Play later
Play later
Lists
Like
Liked
10:24We know what it's like to have a nostalgic moment and be taken back to a memory years ago. The emotion of that memory has a powerful effect on us. While there are a lot of good things about nostalgia, it can influence how a ministry makes decisions if we are not careful.By Jason Lehman
…
continue reading
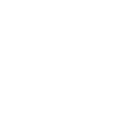
1
21 - Interpretability for Engineers with Stephen Casper
1:56:02
1:56:02
Play later
Play later
Lists
Like
Liked
1:56:02Lots of people in the field of machine learning study 'interpretability', developing tools that they say give us useful information about neural networks. But how do we know if meaningful progress is actually being made? What should we want out of these tools? In this episode, I speak to Stephen Casper about these questions, as well as about a benc…
…
continue reading
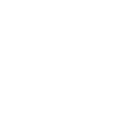
1
20 - 'Reform' AI Alignment with Scott Aaronson
2:27:35
2:27:35
Play later
Play later
Lists
Like
Liked
2:27:35How should we scientifically think about the impact of AI on human civilization, and whether or not it will doom us all? In this episode, I speak with Scott Aaronson about his views on how to make progress in AI alignment, as well as his work on watermarking the output of language models, and how he moved from a background in quantum complexity the…
…
continue reading
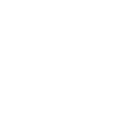
1
Episode 3: E3... An approach to simplify your communication
10:27
10:27
Play later
Play later
Lists
Like
Liked
10:27We've all been there. We are in a moment to share what we do or what our ministry is about and we find ourselves feeling unprepared. The E3 approach is a simple way to structure a presentation into 3 steps that are easy to remember.By Jason Lehman
…
continue reading
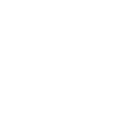
1
Episode 2: Perspective - Our very own vantage point
12:03
12:03
Play later
Play later
Lists
Like
Liked
12:03We all see things a different way. That unique point of view can be a help or a barrier to good communication in your ministry.By Jason Lehman
…
continue reading
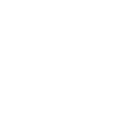
1
Episode 1: An Introduction - The story and heart behind Ministry Edge
12:54
12:54
Play later
Play later
Lists
Like
Liked
12:54Hear from Jason Lehman on his ministry journey, observations about ministry challenges and hopes for Ministry Edge and the tools and resources planned in the coming season.By Jason Lehman
…
continue reading
Store: https://store.axrp.net/ Patreon: https://www.patreon.com/axrpodcast Ko-fi: https://ko-fi.com/axrpodcast Video: https://www.youtube.com/watch?v=kmPFjpEibu0
…
continue reading
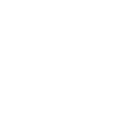
1
19 - Mechanistic Interpretability with Neel Nanda
3:52:47
3:52:47
Play later
Play later
Lists
Like
Liked
3:52:47How good are we at understanding the internal computation of advanced machine learning models, and do we have a hope at getting better? In this episode, Neel Nanda talks about the sub-field of mechanistic interpretability research, as well as papers he's contributed to that explore the basics of transformer circuits, induction heads, and grokking. …
…
continue reading
I have a new podcast, where I interview whoever I want about whatever I want. It's called "The Filan Cabinet", and you can find it wherever you listen to podcasts. The first three episodes are about pandemic preparedness, God, and cryptocurrency. For more details, check out the podcast website (thefilancabinet.com), or search "The Filan Cabinet" in…
…
continue reading
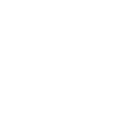
1
18 - Concept Extrapolation with Stuart Armstrong
1:46:19
1:46:19
Play later
Play later
Lists
Like
Liked
1:46:19Concept extrapolation is the idea of taking concepts an AI has about the world - say, "mass" or "does this picture contain a hot dog" - and extending them sensibly to situations where things are different - like learning that the world works via special relativity, or seeing a picture of a novel sausage-bread combination. For a while, Stuart Armstr…
…
continue reading
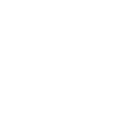
1
17 - Training for Very High Reliability with Daniel Ziegler
1:00:59
1:00:59
Play later
Play later
Lists
Like
Liked
1:00:59Sometimes, people talk about making AI systems safe by taking examples where they fail and training them to do well on those. But how can we actually do this well, especially when we can't use a computer program to say what a 'failure' is? In this episode, I speak with Daniel Ziegler about his research group's efforts to try doing this with present…
…
continue reading
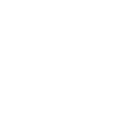
1
16 - Preparing for Debate AI with Geoffrey Irving
1:04:49
1:04:49
Play later
Play later
Lists
Like
Liked
1:04:49Many people in the AI alignment space have heard of AI safety via debate - check out AXRP episode 6 (axrp.net/episode/2021/04/08/episode-6-debate-beth-barnes.html) if you need a primer. But how do we get language models to the stage where they can usefully implement debate? In this episode, I talk to Geoffrey Irving about the role of language model…
…
continue reading
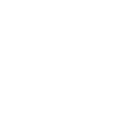
1
15 - Natural Abstractions with John Wentworth
1:36:30
1:36:30
Play later
Play later
Lists
Like
Liked
1:36:30Why does anybody care about natural abstractions? Do they somehow relate to math, or value learning? How do E. coli bacteria find sources of sugar? All these questions and more will be answered in this interview with John Wentworth, where we talk about his research plan of understanding agency via natural abstractions. Topics we discuss, and timest…
…
continue reading
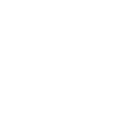
1
14 - Infra-Bayesian Physicalism with Vanessa Kosoy
1:47:31
1:47:31
Play later
Play later
Lists
Like
Liked
1:47:31Late last year, Vanessa Kosoy and Alexander Appel published some research under the heading of "Infra-Bayesian physicalism". But wait - what was infra-Bayesianism again? Why should we care? And what does any of this have to do with physicalism? In this episode, I talk with Vanessa Kosoy about these questions, and get a technical overview of how inf…
…
continue reading
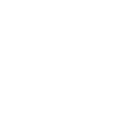
1
13 - First Principles of AGI Safety with Richard Ngo
1:33:53
1:33:53
Play later
Play later
Lists
Like
Liked
1:33:53How should we think about artificial general intelligence (AGI), and the risks it might pose? What constraints exist on technical solutions to the problem of aligning superhuman AI systems with human intentions? In this episode, I talk to Richard Ngo about his report analyzing AGI safety from first principles, and recent conversations he had with E…
…
continue reading
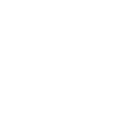
1
12 - AI Existential Risk with Paul Christiano
2:49:36
2:49:36
Play later
Play later
Lists
Like
Liked
2:49:36Why would advanced AI systems pose an existential risk, and what would it look like to develop safer systems? In this episode, I interview Paul Christiano about his views of how AI could be so dangerous, what bad AI scenarios could look like, and what he thinks about various techniques to reduce this risk. Topics we discuss, and timestamps: - 00:00…
…
continue reading
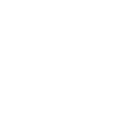
1
11 - Attainable Utility and Power with Alex Turner
1:27:36
1:27:36
Play later
Play later
Lists
Like
Liked
1:27:36Many scary stories about AI involve an AI system deceiving and subjugating humans in order to gain the ability to achieve its goals without us stopping it. This episode's guest, Alex Turner, will tell us about his research analyzing the notions of "attainable utility" and "power" that underlie these stories, so that we can better evaluate how likel…
…
continue reading
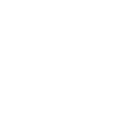
1
10 - AI's Future and Impacts with Katja Grace
2:02:58
2:02:58
Play later
Play later
Lists
Like
Liked
2:02:58When going about trying to ensure that AI does not cause an existential catastrophe, it's likely important to understand how AI will develop in the future, and why exactly it might or might not cause such a catastrophe. In this episode, I interview Katja Grace, researcher at AI Impacts, who's done work surveying AI researchers about when they expec…
…
continue reading
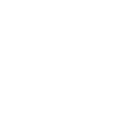
1
9 - Finite Factored Sets with Scott Garrabrant
1:38:59
1:38:59
Play later
Play later
Lists
Like
Liked
1:38:59Being an agent can get loopy quickly. For instance, imagine that we're playing chess and I'm trying to decide what move to make. Your next move influences the outcome of the game, and my guess of that influences my move, which influences your next move, which influences the outcome of the game. How can we model these dependencies in a general way, …
…
continue reading
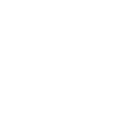
1
8 - Assistance Games with Dylan Hadfield-Menell
2:23:17
2:23:17
Play later
Play later
Lists
Like
Liked
2:23:17How should we think about the technical problem of building smarter-than-human AI that does what we want? When and how should AI systems defer to us? Should they have their own goals, and how should those goals be managed? In this episode, Dylan Hadfield-Menell talks about his work on assistance games that formalizes these questions. The first coup…
…
continue reading
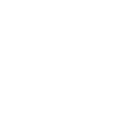
1
Robo Cop (1987) w/ Peter Lehman from Les Merry Chevaliers
2:19:25
2:19:25
Play later
Play later
Lists
Like
Liked
2:19:25Kicking off what I'm calling the post pandemic Season 2 today with a long discussion about Paul Verhoeven's action classic Robocop from 1987 w/ Peter Lehman of Les Merry Chevaliers https://www.facebook.com/LesMerryChevaliers https://twitter.com/merrychevaliers https://www.instagram.com/merrychevaliers Check out LMC's new single Anyhow Anyway at the…
…
continue reading